“Unleashing the Power of Data: Energy Forecasting and Analytics Revolutionizing the Global Energy Sector”
Energy forecasting is the method of applying statistics to make predictions about energy consumption levels and the utility costs associated with them in the short and long term. Energy forecasting is crucial in forming policies related to the global energy market, including demand and supply. Towards this end, data-driven analytics are given importance in the world energy sector, including energy finance and economics.
The big data analytics market in the energy sector is expected to grow at a CAGR of 11.28% during the forecast period of 2022–2027. The impact of big data analytics includes improving energy efficiency and reducing energy consumption.
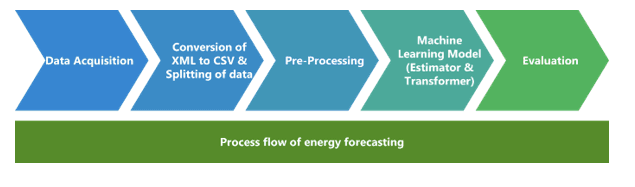
Need of Energy forecasting and Analytics–
The scarcity of fossil fuels, their environmental impact, and the continuous rise in energy consumption have forced governments and corporations to enhance efficiency, optimise processes, and find alternate sources of energy such as solar, wave, and wind turbines.
The volatility in oil prices leads to high expenditures on energy-related projects. Many other problems are related to enhancing efficiency, load distribution, and optimization. Implementation of data analytics helps in predicting product demand, reduces uncertainty, and gives way to resource planning, improved customer experiences, and regulatory compliance.
Data analytics serves as an important component as it provides the necessary data needed for making predictions, performing statistical analysis, and provides prepared data for generating predictive models.
Machine Learning Models-
• Artificial Neural Network (ANN)- A computational model inspired by biological neural networks that mimics the way nerve cells work in human brains. It uses algorithms that can independently make adjustments or learn as they receive new inputs, making it an effective tool for non-linear statistical data modelling.
• Support Vector Machine (SVM)- A type of deep learning algorithm that performs supervised learning for regression or classification of data groups. The classification offers a learning basis for future data processing. Algorithms separate groups according to patterns.
Machine Learning Applications to Energy Forecasting and Analytics-
- Accurately predict energy prices- It helps to predict energy price changes by analysing tiny changes in the thousands of factors that affect energy prices
- Accurately predict energy demand- It could predict the energy demand by analysing various factors that affect it, like the day of the week, time, major sports event, air temperature, past demand, and mean demand
- Optimize energy consumption- With the help of smart meters, IOT devices, and non-intrusive appliance load monitoring (NIALM), a machine learning algorithm can be used to identify the energy consumption at the device-specific level
- Predict Customer Lifetime value- CLV helps utility markets identify how much any given customer is going to spend over the term of their contract. Machine learning can predict the overall value of an individual customer
- Optimize prices through better trading- To stay competitive, where customers have a choice in the provider of their electricity, machine learning can provide information that affects energy prices and provides the energy when to buy and sell it
- Reduce customer churn- To avoid customers switching to another provider, it becomes imperative to identify and prevent customer churn. Machine learning techniques such as the Cross-Industry Standard Process for Data Mining can be used
- Probabilistic Forecasting- Summarises opinions, what is known, and future events. Instead of providing single-value forecasts, probabilistic forecasting assigns a probability to various outcomes, and the complete set represents a probability forecast
- Load Forecasting- Is a technique used to predict future energy consumption to meet demand
- Electricity Price Forecasting- A Branch of Energy Forecasting Focused on the Spot and Forward Prices in Electricity Markets
- Wind Power Forecasting- This provides data on how much wind power is expected at a particular instant of time in the days to come
- Solar Power Forecasting- Process of gathering and analysing data to predict solar power generation on different time horizons
Conclusion-
Machine learning has become a tool for the energy sector that is able to solve problems and arm the sector with solutions and information by analysing large quantities of data, almost touching every aspect of the industry from optimising prices, increasing efficiency, reducing uncertainties, identifying and forecasting demand, and various other factors, allowing the energy sector to prepare themselves for the present and future requirements and challenges faced by the sector and providing support to the renewable energy sector too.
Author: Abhishek Saini